Plant diseases threaten global food security by reducing crop yield, so diagnosing plant diseases is critical to agricultural production. Artificial intelligence technologies gradually replace traditional plant disease diagnosis methods due to their time-consuming, costly, inefficient, and subjective disadvantages. As a mainstream AI method, deep learning has significantly improved plant disease detection and diagnosis for precision agriculture. In the meantime, most of the existing plant disease diagnosis methods usually adopt a pre-trained deep learning model to support diagnosing diseased leaves. However, the common-used pre-trained models are from the computer vision dataset, not the field of botany, which hardly makes the pre-trained models have sufficient domain knowledge about plant disease. Furthermore, this pre-trained way makes the final diagnosis model more difficult to distinguish between different plant diseases and lowers the diagnostic precision. To address this issue, we propose a series of commonly used pre-trained models based on plant disease images to promote the performance of disease diagnosis. In addition, we have experimented with the plant disease pre-trained model on plant diseases diagnosis tasks such as plant disease identification, plant disease detection, plant disease segmentation, and other subtasks. The extended experiments prove that the plant disease pre-trained model can achieve higher accuracy than the existing pre-trained model with less training time, thereby supporting the better diagnosis of plant diseases.
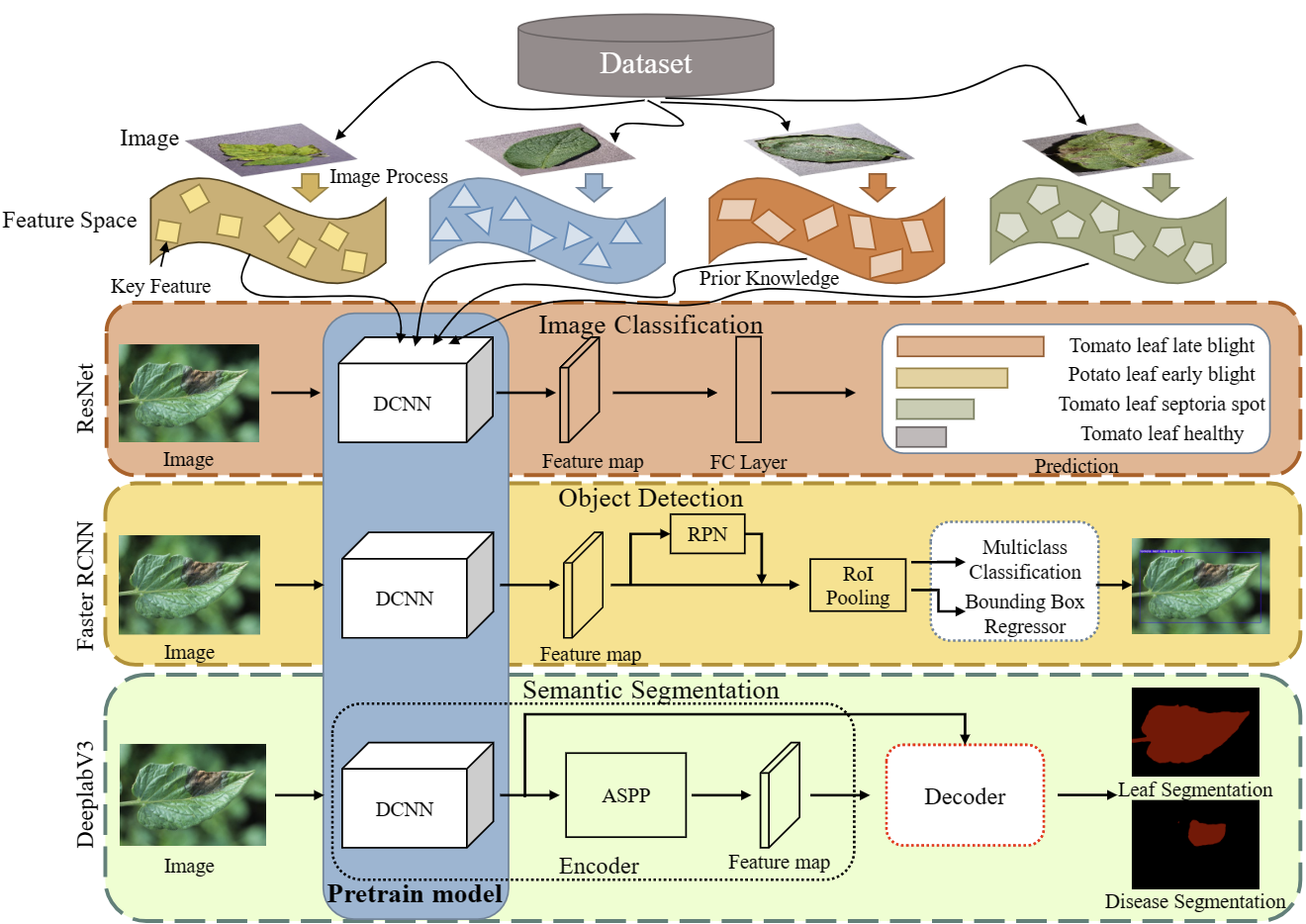
The role of pre-trained models in plant disease diagnosis